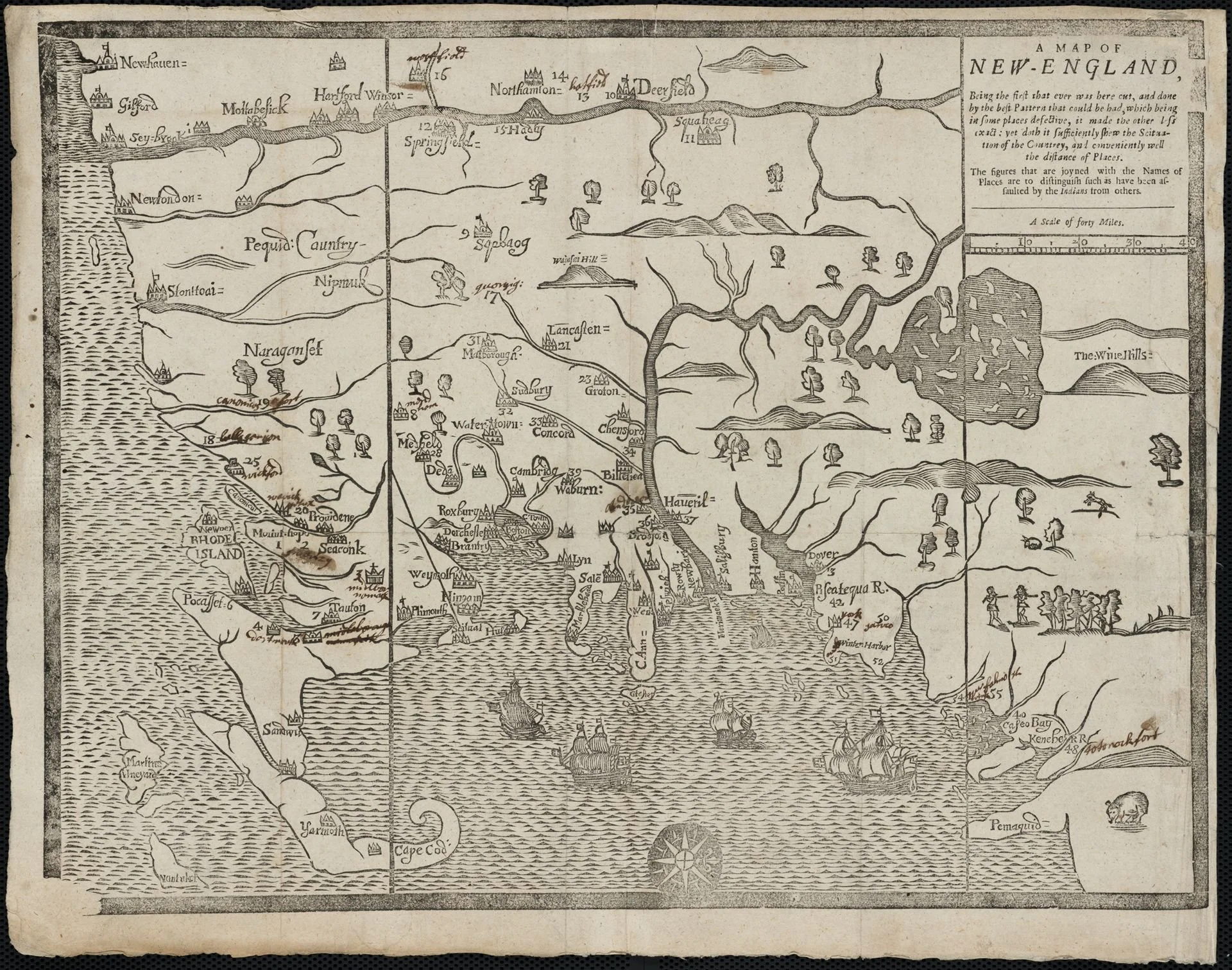
James P. Freeman: Applying data analysis to Supreme Court nominees
U.S. Supreme Court Building.
Last autumn, techrepublic.com concluded, with its feature “How big data won the 2017 World Series,” that America’s pastime was more the cold science of analytics than the graceful art of, say, a George Springer swing. This fall, progressives hope that big data will win the day to thwart Judge Brett Kavanaugh’s ascension to the Supreme Court.
When the book Moneyball: The Art of Winning an Unfair Game was published in 2003, it acted as a catalyst for Major League baseball teams to “start taking data-based decision making more seriously.” The employment of metrics was also rooted in cost-effective ways to win. The prize was the Fall Classic.
Baseball, already known for its rich sediment of data, hired experts to “make data-driven decisions based on predictive analytics.” Perhaps the biggest manifestation of data predicting outcomes is the use of “the shift” — a technique where a coach will move his defensive players to one side of the field knowing, in advance, that a hitter will put a ball into play there a statistically significant number of times. (Shifts increased from 2,350 in 2011 to 28,130 in 2016). TechRepublic notes that teams with a “prowess with data” — the once moribund Boston Red Sox, Chicago Cubs and Houston Astros — are recent World Series champions.
Some now believe that these methodologies can be applied to Supreme Court nominees.
Data for Progress is, in its own words, “the think tank for the future of progressivism.” Claiming that “a new generation of progressives is rising,” it wants to be the Elias Baseball Analyst for left-leaning causes. Using scientific techniques to support progressive activists and issues, it also aims to “challenge conventional wisdoms about the American public that lack empirical support.”
Areas of its research include: “Multilevel Regression and Poststratification analysis,” to provide “reliable sub-national opinion estimates on progressive issues;” “deep learning textual analysis of media;” and “data mining and analyzing social media data for politicians and pundits to find interesting trends and patterns.”
Pitchers and catchers beware!
In another sign of the miniaturization and mobilization of complex matters on social media, Data for Progress prefers to distribute its research over the internet because “data can only help interpret the world.” (Many credit its co-founder, Sean McElwee, with inspiring the “Abolish ICE” movement based upon a tweet he posted in early 2017.)
Today, however, McElwee is focusing on the Supreme Court. And his opposition to Judge Kavanaugh.
Data for Progress believes that a Kavanaugh seat on the high court would set back progressive causes in areas such as voting rights, Medicaid, corporate pollution, unions, gerrymandering, and, most urgently, abortion rights.
A key component of its approach is that ideological implications can be measured. Data for Progress uses a political science methodology called Judicial Common Space, which seeks to answer, among other things, why courts make the decisions they do. Judicial politics, like a Chris Sale slider, can be measurable and explainable. Or can it?
Justices are “scored” or measured in similar ways that members of Congress are measured for their roll call votes. So, Justice Sonia Sotomayor appears on the left of the spectrum and Justice Clarence Thomas appears on the right. But, like all computer modeling, how Kavanaugh fits into the equation — hence, how he would affect the ideological balance of the court — is entirely hypothetical.
Data for Progress suggests that judicial reasoning is necessarily a linear process; past judicial decisions are a measurable and definitive predictor of future decisions. This in turn determines where judges stand on an ideological plane.
For Data for Progress — even if its quantitative analysis stands close scrutiny — prospective liberal justices are acceptable and conservative justices are not. Big data is now political fodder for modern progressives.
But history may prove McElwee and Data for Progress wrong.
In a recent piece for The Nation, McElwee argues that “Democrats Must Stop Pretending the Supreme Court is Apolitical.” He worries about the looming “threat” of a Supreme Court that could “reverse progressive legislative accomplishments.” When did Democrats stop believing the court was apolitical?
In 1937, President Franklin D. Roosevelt, a Democrat, attempted a ''court-packing” plan. His motivations were entirely political. He intended to shape the ideological balance of the court so that it would cease striking down his New Deal legislation. And in 1987, Democrats launched a full partisan attack on the nomination of Robert Bork to the high court. The conservative jurist was soundly defeated over what conservatives believe were purely political motivations. There is a long history of partisan battles in picking Supreme Court justices.
And Data for Progress’ fears of the court — and, by extension, the nation — being held hostage by conservative justices for years to come may be mistaken. In fact, big data may prove Data for Progress to be just another political lever of the progressive organ. And render their rantings moot.
Fivethirtyeight.com concluded three years ago that “Supreme Court Justices Get More Liberal As They Get Older.” The “ideological drift” of justices is cited in a 2007 academic paper. The authors of the study, using scores based on data from the Supreme Court Database, write that: “Drift to the right or, more often, the left is the rule, not the exception.”
A 2005 New York Times expose, “Presidents, Picking Justices, Can Have Backfires,” should be a reminder to Data for Progress that, despite a wealth of data, Supreme Court justices’ ideology is malleable and subject to change. As President Eisenhower learned of Chief Justice Earl Warren and President George H.W. Bush learned of Justice David Souter.
Whatever their political persuasions, Americans are now keeping score in two spectator sports because of big data.
James P. Freeman is a former banker and now a New England-based essayist. This piece first appeared in Inside Sources.